In May 2022, the Finnish public broadcaster Yle noticed an audience they weren’t reaching. Tens of thousands of Ukrainians displaced by the war had moved to Finland. Yle offered news in Finnish, Swedish, English, and Russian. Now, they wanted to offer it in Ukrainian. But it wasn’t easy finding Finnish journalists who could speak the language.
“When the war started, every media company was interested in those people,” says Jarkko Ryynänen, project manager for the Yle News Lab. Instead of having staff rewrite stories in a new language, Yle turned to software to translate. The team built a tool that ran stories through four different types of translation software and presented the results to staffers who knew both languages. It allowed Yle to publish stories in Ukrainian at a rate that would be impossible if the news were written and reported in the language from the beginning, the way many articles for Yle’s Russian and English services are. “With the computer, this couple of people are so much more powerful,” Ryynänen says.
Yle using AI to translate its articles is one of the more upbeat stories about artificial intelligence (AI) in newsrooms in a time that’s full of dire predictions for the technology’s impact on journalism.
The public launch of image generators such as DALL-E and Stable Diffusion and the chatbot ChatGPT had users flooding social media with machine-generated words and pictures that were crafted so well that the results seemed nearly authentically human. We’re used to seeing computers work with highly structured data like spreadsheets or lines of code, but these apps process words in a highly complex way. They offer a glimpse of how AI could both replace human writers and fill the internet with false words and pictures, thus making the role of a reporter simultaneously obsolete and more necessary than ever.
Early experiments stoked these fears. The tech news website CNET announced earlier this year that it was pausing its program to write stories using AI after the resulting articles were not only riddled with errors, but rife with plagiarism. Mathias Döpfner, CEO of German Publisher Axel Springer, which recently acquired Politico, wrote in a company email in February that “artificial intelligence has the potential to make independent journalism better than it ever was — or simply replace it.” In January, Buzzfeed announced internally that it would use technology from OpenAI, the company behind ChatGPT, to automate the writing of its signature quizzes. To assuage concerns, a spokesperson said the site “remains focused on human-generated journalism.” Two months later, Buzzfeed began publishing travel articles written with AI. Shortly after, it announced the end of its Pulitzer-winning news operation.
“Human-generated journalism” can be hard to define. Visit a newsroom today and you’re likely to find reporters uploading recordings of their interviews to transcription services like Otter or Trint, while social media editors track trending topics using algorithms from Crowdtangle. A study from the Reuters Institute found that two-thirds of surveyed newsrooms were using AI to customize readers’ experiences, for instance by recommending stories they might like to read. Before it tried translations, Yle began using AI to track lawmakers’ votes. Another example is The Associated Press, which has published machine-written financial stories since 2016 with a goal of giving reporters time to focus on in-depth reporting.
AI follows search and social media in promising further disruption to the news business —especially as social media networks struggle to maintain relevance and search engines increasingly add AI features and chatbot interactions to their services. Google announced a new service this spring that would respond to some search queries with an AI-generated response instead of the usual list of links.
Cleverly coded chatbots may threaten to reinvent the way we write news, but AI in other forms isn’t so much replacing journalists’ jobs as it is automating so many of the tasks that were heaped onto their workloads over the last 20 years. AI is helping newsrooms reach readers online in new languages and compete on a global scale. It’s studying publishers’ stories to find patterns in reader behavior and using those patterns to serve readers stories they’re more likely to click on. AI is even filling in boilerplate paragraphs and giving writers a head-start on their first drafts.
“If we think of AI as a support tool in a lot of these various arenas, we get a lot more mileage out of what AI can actually do,” says Dalia Hashim, AI and media integrity lead at the Partnership on AI, a nonprofit coalition of businesses, media, and academic organizations that recommends best practices for using AI.
But the difference between being helped by AI and being replaced by it lies in understanding how it works and reinforcing the value of the human side of journalism.
AI, Natural Language Processing, and Journalism
“Artificial intelligence” is something of a catch-all for advanced algorithms that power everything from web searches to the alerts you get on your phone encouraging you to look at old pictures to more advanced applications like DALL-E and ChatGPT. Both DALL-E and ChatGPT, which stands for Generative Pre-Trained Transformer, are made by the same company, OpenAI. Generative refers to the technology’s ability to generate data — words or pictures in these cases. Pre-trained refers to the process of feeding the app sets of data to inform it. Transformer is a type of machine learning that processes that data.
Essentially, it studies billions of examples — say a library of text — and then produces an output based on a prediction of what’s likely to come next.
Ask ChatGPT to describe a bluebird and it will tell you that it’s a small bird with blue feathers, among other facts. Put simply, this answer is the result of computations that check the data the app has been trained on for patterns that match the question posed to it. The program then synthesizes a response based on a machine-powered understanding of the order letters and words go in. If none of the data ChatGPT was trained on included anything ornithological, it would come up short.
ChatGPT’s training data includes nothing from later than September 2021. Ask it what happened in 2022 and it will give a disclaimer that it doesn’t know, then list a few safe predictions — “climate change continued to be a major issue” — and scheduled events like the Beijing Olympics. Tools like this employ, or fall under the category of, natural language processing (NLP) — AI trained on datasets of human language so it knows not only what a verb is, but where to place one in a sentence, and how to conjugate. This can make the technology feel especially competitive to humans, since it enables computers to communicate in ways we clearly understand. That communication is often quite good and keeps improving.
A chatbot’s writing ability can be impressive, but much of NLP’s power for newsrooms lies in its ability to read. NLP tools can analyze text at a scale beyond human capability. And as Yle and other newsrooms are finding, having a trained machine reader can lead to more human readers as well.
Translation
Translation is one application of NLP: It may not be the most widespread or most well-publicized, but it shows the power of AI to increase reach and revenue — and also the pitfalls of relying too heavily on machines.
A month before Yle posted its first stories in Ukrainian, French newspaper Le Monde launched its English-language edition. The project began when a reporter proposed writing a series of articles on the 2022 French elections in English. Knowing the efficiencies of machine translation, Le Monde considered instead launching a newsletter that would offer 10 to 15 of the more than 100 articles the paper publishes every day translated into English. But given the capacity AI provided, the paper quickly shifted to launching an entire English language edition.
Now, the first element at the top of Le Monde’s homepage is a button to choose between French and English. The English edition offers 40 articles every weekday and 30 each day on Saturday and Sunday. Like Yle’s Ukrainian stories, Le Monde’s English posts are written and edited in the outlet’s native language, then translated to English with AI, and reviewed by an editor before publishing. The process is called machine translation post-editing (MTPE), and it enables a small group of humans to do the work of many.
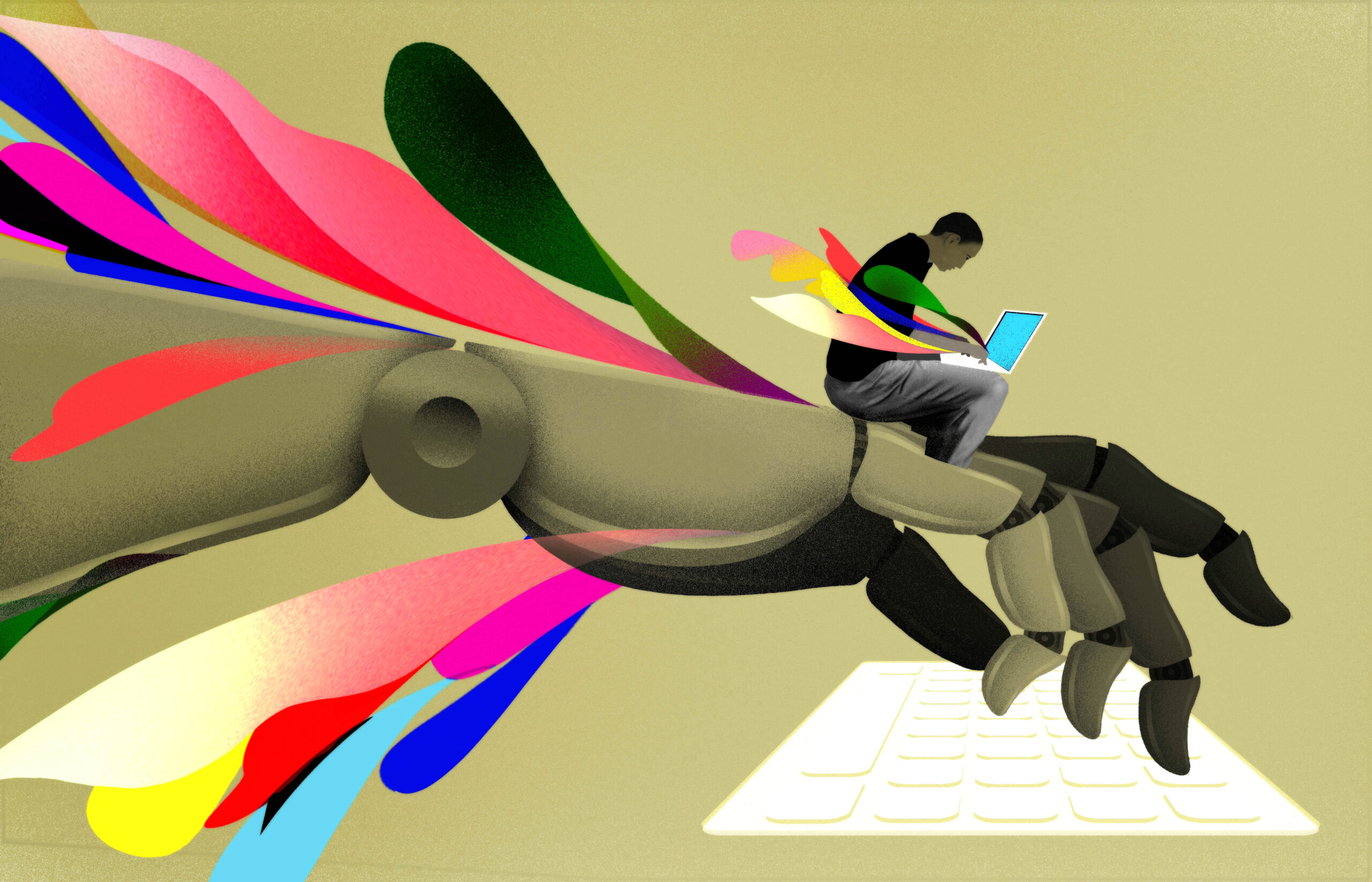
Brian Stauffer
“If there were only the seven journalists currently working on the project writing [English-language] stories, we would publish maybe one to five” a day, Le Monde English editor Elvire Camus says. But using AI doesn’t mean the process is easy. The paper takes several human-centered steps to not only ensure the translations are accurate, but to check context, cultural literacy, and preserve the editorial style Le Monde has been building for about eight decades. “The system is quite heavy,” Camus says.
Le Monde partners with two outside firms that specialize in MTPE to undertake these translations, one of which is TranslationServices.com. When Translation Services gets its articles from Le Monde, it runs them through DeepL, an AI translation program. This tool doesn’t just translate word-by-word like a human flipping through a dictionary. It connects phrases and context among words. But the tool isn’t yet advanced enough to produce translations that Le Monde would run without review. “We want to make sure that what we publish in English is as good as what we publish in French,” Camus says.
The post editors Translation Services employs are veterans of government offices or French literature scholars who do more than check that the translation is technically correct. There are punctuation discrepancies and cultural differences, like describing French municipalities. In addition, “the literary flair is lost, often,” says Luke Palder, chief executive officer of Translation Services, and certain concepts might need more explanation for an audience outside of France. “You would never explain who Nicolas Sarkozy is in French, but to an English audience, you would sometimes need to say he’s the former president,” Camus says.
Le Monde has a stylebook for translators that explains these differences and helps streamline the review of machine translations. “The style guide has grown and grown,” Palder says. “And that’s really reflective of the fact that AI helps us get the job done, but at least for the foreseeable future, there is always going to be … a human in the loop, as machine learning folks sometimes say.”
After DeepL and the post editor, a story gets one more review by a Le Monde editor. By the time a story lands on the English homepage, it’s been through four pairs of eyes and one AI program, Camus says.
Translation Services tries to keep the same people on the team editing Le Monde — which is spread around the world to respond to news at all hours — because humans get more efficient the more they work with the machines. “There’s almost this machine learning type approach to the work that we’re doing, where the translators themselves start to understand the ways in which the AI is likely to have messed up,” Palder says. Likewise, the machines learn from these corrections. Some repeat issues can be coded into the translation software, so the machine knows to translate a certain idiom a certain way, or to space em dashes appropriately.
This ability to adapt and be retrained is one reason why even the free and instant translations available online have become better over the years. And the more uses of language we employ, the more AI has to learn from.
Look through online translation services and the most commonly available languages will be ones with large bodies of training material — that is, an abundance of texts in overlapping languages. AI translation tools are just starting to crack languages that haven’t been widely published, or widely published in multiple translations. This includes indigenous or near-extinct tongues. “There’s really no reason that news should be siloed by language,” Palder says, noting there may come a time when news outlets don’t feel the need to have a human review of translations.
Le Monde’s goal is to make the English edition a source of revenue. The paper hopes to have 100,000 English-language subscribers by 2025. Camus declined to share current subscriber numbers but said growth for the English service has been steady, if modest. The name Le Monde carries more weight in the French-speaking world; launching a translated service aimed at readers with no previous relationship to the paper is like launching an entirely new publication, albeit one with a much larger potential audience. English is the most-spoken language in the world; there are over a billion more people who understand it than understand French. Le Monde sees its competitors as the BBC, The New York Times, and The Washington Post. AI’s efficiency has enabled them to begin to compete with these other global, English-language publications in terms of scale.
Yle has encountered a similar challenge finding an audience for its Ukrainian news. They have some readers, but not as many as they hoped. Samuli Sillanpää, tech lead at the Yle News Lab, says this may be because the audience they’re after would rather get news in a different format. Fortunately, AI can also be used to translate audio and video stories, and Yle is looking into implementing it.
Text Analysis
Many reporters may already be using AI language analysis that’s a little more rudimentary than ChatGPT and less complicated than MTPE. Apps like Grammarly, for instance, scan texts for grammatical errors and suggest ways to avoid phrasing that’s technically correct but might be stylistically undesirable, such as passive voice or awkward syntax.
By using AI tools to analyze news articles, rather than translate or write them, journalists can gain a better understanding of the work they do and help get that work to readers.
In 2022, the staff at The Atlanta Journal-Constitution saw an opportunity to rework the email newsletter they send to subscribers in the afternoon. “We had this hypothesis that maybe that would be a great place to provide some personalized recommendations for folks and give them a mix of engaging and essential stuff to read,” says Zack McGhee, senior director of digital audience experience for the paper. That personalization, they decided, would be done by an AI, while the paper’s other newsletters were curated by editors.
“We believe that automated ways for analyzing our content are important for building effective methods that can guide our editorial processes” — Samuli Sillanpää, tech lead at the Yle News Lab
For automation, the paper turned to the Belgium-based firm Twipe, which offers publishers newsletters that are curated and sent entirely by a machine. Twipe’s software works by using an AI to assign categories to a publisher’s content. In many newsrooms, this is done by humans —the person who loads a story into a content management system assigns tags and categories based on their reading of the text. These tags are helpful not just for recommendations, but also to determine how popular a particular topic is. But these tags can also be inconsistently applied. A story on a soccer match might be tagged “sport” while another soccer story is tagged “sports.” Twipe’s AI reads hundreds of stories and groups them into categories based on patterns of how words are used, finding trends that might elude a human editor or go overlooked in a manual tagging process.
In addition to studying a publisher’s archive, Twipe analyzes the stories a reader has clicked on while logged into their account. It then matches that user’s history to its machine-created topics. The resulting newsletter is a mix of these recommendations and stories that are trending more broadly across the publisher’s website. “That ensures that you don’t go into things like filter bubbles, because you’re still getting the same main stories of the day or the key stories that you should know, alongside things which are kind of personal interest stories,” says Matthew Lynes, former content marketing lead at Twipe. (Lynes left the company in March.)
Currently, some 50,000 Atlanta Journal-Constitution subscribers are getting a daily afternoon email that’s been put together and sent entirely by AI, with stories based on their reading history. McGhee says another 50,000 are getting human-curated newsletters. The open rate for the human-curated newsletters is about five percent, while Twipe’s emails are six-and-a-half percent. It’s small, McGhee acknowledges, but that number has grown over the six months they’ve tested the emails. And for McGhee, the more encouraging statistic is how engaged readers are with the automated emails. “We’ve seen about 600 people become more activated,” he says. This means they more regularly open emails and more often click on stories — reading at least 10 per month.
This incremental growth is the result of careful human oversight of the AI. Even though Twipe’s software can send thousands of custom emails automatically, a team of Journal-Constitutionand Twipe staffers closely monitor the project. Dana Nastase, Twipe’s head of business development and incubation, says there’s typically a learning period for the AI and for its users — newsrooms may decide to surface more local stories or to not include editorial cartoons or very short stories. It’s a lot of work for something that, by design, could be fully automated one day, or done by a human editor who can choose which stories readers should see. But McGhee says the customization of stories is one way to ensure the newsroom is reaching as wide of an audience as possible. “We have a lot of different audiences, folks across generations and genders and the like, who we want to reach,” he says. “We can serve up content that is interesting and exciting and relevant and essential to them.”
AI’s categorizations don’t need to stop at topics. The startup Overtone AI has created models to study the relationships between words and paragraphs, and its software can identify how much opinion, original reporting, or aggregation articles include. “Is this a quick update about a car crash with one source that is the police, or is this an in-depth investigation about car crashes that speaks to everyone in the community about how a particular intersection is ruining their lives?” is an assessment the tool can make, says Christopher Brennan, chief product officer for Overtone. The software gives stories a score that predicts their level of impact. Publishers can then compare that score to their existing analytics to get a sense of not only what topics readers engage with, but what type of story.
“People can understand one article now, anecdotally with their intuition of saying, ‘Ah, this sort of article did well on social or this sort of article led to newsletter signups,’” Brennan says. “When you have the help of an AI, you can look at hundreds or thousands of articles, and then see, okay, consistently, we see one type of article leads to newsletter subscriptions, or one type of article is what leads to increasing our reach on social.”
The Institute for Nonprofit News (INN) — a collection of more than 400 independent newsrooms across the country — uses Overtone to sort the hundreds of stories its partners publish every day. This sorting is helpful for publishers in the collective who might want to share a partner’s work with their audience, but don’t have the time to read every story. The AI categorizes stories by theme and format and presents a short list of pieces that fit a publisher’s criteria for sharing.
“It’s the first cut at curation,” says Sue Cross, executive director and chief executive officer of INN. The technology winnows down a list of hundreds of stories to a handful in a fraction of the time it would take human to do so.
In one instance, Overtone labeled a Texas Tribune story on a shortage of maternity care in East Texas as an in-depth enterprise story about rural issues. In early January, INN featured it as its story of the week in its newsletter, putting it in front of readers outside of Texas and other news organizations that might then share the story with their audiences. This kind of sharing “extends the impact of this coverage, and it helps with distribution of audience funnels,” Cross says.
INN isn’t eliminating human curators, though; it’s just giving them some help, she adds.
Source and Fact Checking
This kind of analysis has uses beyond aligning stories with reader interests. Yle’s engineers are exploring how AI could scan stories to look for signs of bias or identify blind spots in diversity. This begins by tracking sources’ demographic information, backgrounds, and political affiliation. Yle “currently track[s] some attributes manually,” says Yle News Lab’s Sillanpää, “but this is understandably time consuming and doesn’t scale.” By next year, Sillanpää hopes to have AI-powered source-tracking at Yle. Combined with other analytics, this means the newsroom would be able to see who is quoted on which issues, with what frequency, and in what type of story.
This super-charged source audit could go even further, by deploying an application of NLP called sentiment analysis. This is a way that AI spots tone by examining the relationship between words. Some newsrooms use it to alert editors of hateful or aggressive posts in comment sections. Sillanpää says combining it with topic tags and source tracking would lead them “to figure out what kind of terminology is used to discuss certain topics,” possibly identifying unconscious bias in stories.
Sillanpää predicts AI will eventually scan video and audio stories, too, and give Yle’s journalists a more granular view of not only of what they cover, but how. “The main idea is to make our stories better,” Sillanpää says. “We believe that automated ways for analyzing our content are important for building effective methods that can guide our editorial processes.”
Scanning with NLP tools doesn’t need to be limited to content newsrooms produce themselves. This is a use that’s bound to become more vital for journalism as AI grows as a means of generating content online. Kristian Hammond, cofounder of Narrative Science, a company that trains AI to write news, has predicted that 90 percent of news could be written by AI by 2030.
That number is a prediction, but AI-generated content is already filling up our feeds, whether it’s in the form of social media filters that alter our appearance or chatbots that respond to customer service questions. The science fiction journal Clarkesworld closed its submissions portal in February 2023 after editors were flooded with hundreds of short stories written by machines. Some of this AI content is for fun or is used in the name of efficiency. But other examples are meant to misinform or to profit from clicks in the attention economy.
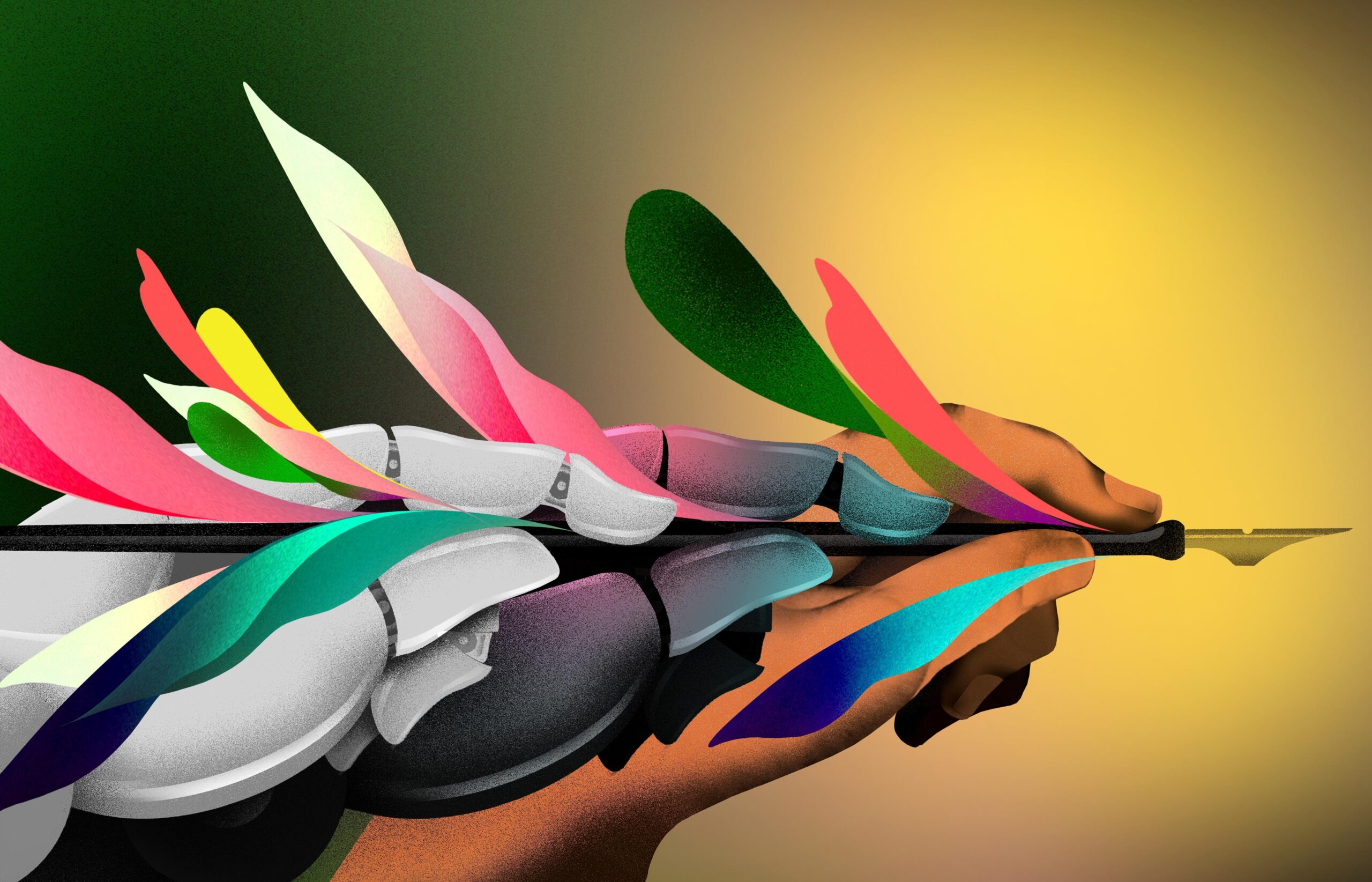
Brian Stauffer
Here, the best tool to fight misleading AI could be AI itself — a tool programmed to recognize images or text created by another machine. Earlier this year, a college student named Edward Tian released an app called GPTZero, which assesses the probability that a text was written by AI. The tool has applications in classrooms, where teachers might use it to check for students cheating on essays, but Tian has opened it to anyone. A user simply pastes text into a website to get a score of how likely it was the text came from a machine.
Programs like GPTZero work by essentially measuring the complexity of sentences and the range of word choice in writing. AI tends to repeat patterns, and AI detectors look for those. They’re not entirely accurate and do generate false positives, but they can be useful tools in identifying text that may be partially or entirely written by a machine.
As we’re inundated with automated information, it’s tempting to think AI could go a step further and recognize misinformation, whether it’s made by a human or a machine. But pure fact-checking is unlikely, at least in the sense that we might imagine AI labeling every statement true or false. To do this, a fact-checking AI would need to be trained on a database of every known fact, and even then, it couldn’t detect falsehoods like fabricated quotes.
Instead, Brennan believes NLP could be deployed to look for language patterns that are commonly found in misinformation — patterns like “hyper-partisanship, lack of sources, vague assertions, toxicity.” Tools like this can sort through the flood of online content and identify misinformation, AI-written text, and deepfakes at the same speed at which they’re created, which is much faster than any human fact-checker could operate. This would be helpful in steering reporters who want to debunk misinformation, or it could be deployed to reduce the financial incentive for posting eye-catching but incorrect information. Brennan envisions pairing this type of tool with advertising software so advertisers could avoid having their messages appear next to likely disinformation or deepfakes.
Stories Written by AI
Not all AI writing is bound to be misinformation or inaccurate. Thousands, likely millions, of news consumers have already been informed by AI-generated stories. The Associated Press uses AI to write updates on corporate earnings reports and sports stories. The BBC has experimented with AI for local election updates. Yle briefly used an AI to write sports stories.
AI can write these types of stories because they’re based on known events that produce clear data: Hockey games end in a score, corporations routinely file financial updates, vote tallies are posted publicly. Humans are good at writing these stories, too, but writing them takes time that could be put to use on enterprise and investigative stories — the type of pieces that don’t have clean, publicly posted results.
AI writing doesn’t need to be an all-or-nothing proposal, though. The more tedious parts of the writing process for more involved stories can be done by machines in a process that gives humans control over the final product. Narrativa, a natural language generation company that works with publishers, created the technology behind a tool The Wall Street Journal uses to help draft stories for reporters by putting together paragraphs on market trends and financial data. “From that starting point, a reporter can layer on analysis and insight — producing a richer story faster,” Alyssa Zeisler, then The Journal’s research and development editor, wrote in 2021. “With the computer focusing on the ‘what,’ our reporters can focus on the ‘why.’”
Certain precautions need to be taken. An AI trained on information from across the internet may not have the right information to draft a Wall Street Journal story, and it certainly won’t write like a Wall Street Journal reporter would write. Narrativa’s tool is trained on data from Dow Jones and the Journal’s archives — the paragraphs it writes have the paper’s institutional voice. This also eliminates the question of ownership for a story written by a machine that’s trained on the work of tens of thousands of different people. “Newsrooms should use only their archives if they want to produce their own content and own the [intellectual property],” says David Llorente, Narrativa’s CEO. (OpenAI’s terms require anyone publishing text made with its tool to label it as such.)
Even with this training, Llorente says, AI is best left to helping with paragraphs, not whole stories. “Language models are not knowledge models, and they should never be used to write stories but rather to help journalists with some tasks,” he says. “People tend to think that models like GPT3 or ChatGPT can be used to write news, and this is not possible. These models are great to perform traditional natural language processing tasks such as summarization, paraphrasing, [and] information extraction.” This is because ChatGPT and others work like aggregators — only finding what already exists, not what has yet to be uncovered. It’s why Narrativa can build an AI that writes a paragraph based on public data, but it can’t report out a new story or find information that hasn’t been posted publicly.
“The more we understand how those tools operate and the less of a magic box it seems to the people involved, the more we’re able to make some of these decisions in an informed fashion” — Dalia Hashim, AI and media integrity lead at the Partnership on AI
Another use for AI in the newsroom could be to summarize stories in order to distribute them in news briefs, newsletters, social media, or on other platforms. This may become more widespread soon. In a survey of local newsrooms in published 2022, The Associated Press found that summarization was among the most-requested AI tools, and the survey mentioned that the AP’s partner, the AI firm Agolo, could not only summarize text, but turn text articles into broadcast scripts, a task many multi-platform newsrooms dedicate several human hours to every day. In these instances, the AI is deployed after a human has reported and written a story. It doesn’t replace the reporter but rather reduces one part of their workload.
The AP survey shows that many smaller newsrooms want AI to take on those tasks that take away time from human reporting but are essential to finding an audience and giving that audience news they’re likely to want. These tasks include summarization not only of stories, but also of transcripts of government meetings. It includes adding metadata to photos and stories, transcribing interviews and videos, writing closed captions, and so many other jobs that have become chores in journalism’s digital age.
But even if it might make their lives easier, the threat of AI is still top of mind for many journalists, especially as AI’s ability to help draft stories improves. Some publications have set rules around it, like Wired, which published a list of ways it would or would not use AI. The publication said it would not publish text written by a machine, though it might use AI-suggested headlines or social media posts. It was reported in April that Insider was forming a working group to experiment with using AI for everything from story outlines and interview questions to search-friendly headlines and copy editing. As AI advances, its potential to remake newsrooms grows, and so do the risks for journalists who may see AI as a passing trend or an existential threat, rather than a new set of tools.
At Yle, the initial adoption of AI was met with skepticism. “Every time we introduce something like that the first or second question will be: Will this robot sack me?” Ryynänen says. The answer was to frame AI as a colleague, not a replacement. It started with giving the AI a name — Voitto — and representing it with a cartoon of a robot. “We made up this doll and this figure Voitto and somehow it worked,’” he says. If “he’s not so dangerous looking then there’s nothing to be feared.” Silly as it may seem, this façade helps journalists get comfortable working with a new set of tools.
That process starts like it did at Yle, by learning what AI can and can’t do, understanding that it carries the biases of the people who made it, and recognizing that it does the work people ask of it. “The more we understand how those tools operate and the less of a magic box it seems to the people involved, the more we’re able to make some of these decisions in an informed fashion,” says Hashim, the AI and media policy researcher, “as opposed to thinking of an AI as a one stop shop tool that … solves everything.”