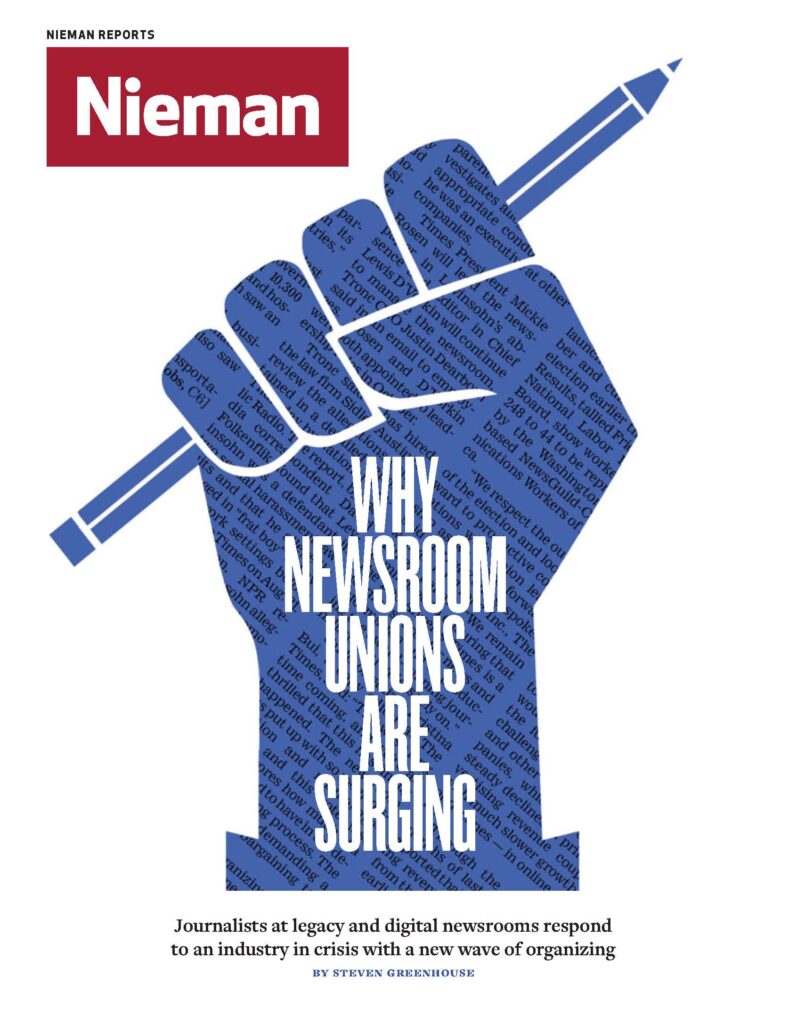
When Gawker employees voted to unionize back in 2015, it was the first major news site to take that step. The now-defunct outlet’s move sparked a movement, with a wave of unionization taking place at both legacy and digital newsrooms across the country.
This surge comes as a bright spot in a labor movement that has been declining for decades—and as the media industry is in crisis. Labor’s supporters say the industry’s volatility began long before the surge of organizing, and that unionization actually improves publications. Journalists are saying “yes” to unions to lift salary floors, win or improve basic benefits, and provide some cushion during a time of uncertainty.
Nicholas Diakopoulos, director of Northwestern University’s Computational Journalism Lab, is optimistic about the role algorithms can play in the media, but he acknowledges that ensuring their ethical use will require vigilance. Bots with nefarious aims make a lot of headlines. This excerpt from his book “Automating the News: How Algorithms Are Rewriting the Media,” published June 10 by Harvard University Press, focuses on bots with a public-spirited and/or accountability purpose:
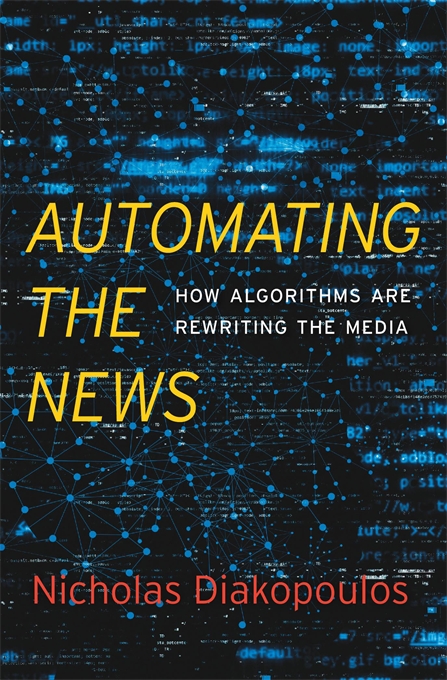
In just onemonth in 2017 an unpretentious little bot going by the handle “AnecbotalNYT” methodically pumped out 1,191 tweets addressed at news consumers on Twitter. It’s perhaps surprising to see people genuinely engage the bot—a software agent that presents itself as nothing more—replying to or agreeing with it, elaborating on the views it curates, responding emotionally, rebutting or explicitly disagreeing with it, even linking to contradictory videos or articles. Eighty-eight percent of the replies were from the user the bot had initiated contact with, but 12 percent were actually replies from other Twitter users. By catalyzing engagement both with the targeted user and with others who could then chime in, the bot opened the door for human users to interact more with each other.
I designed AnecbotalNYT as an experiment to help raise awareness for interesting personal experiences or anecdotes written as comments to New York Times articles. It works by first listening for tweets that have a link to a New York Timesarticle. Then it harvests all the article’s comments, scoring each text based on metrics such as length, readability, and whether it describes a personal experience. The comments are ranked by an overall weighted score, and the bot selects a comment likely to contain a personal story or anecdote. The selected comment is then tweeted back at the person who had originally shared the article link. If the person was interested enough to share the link on Twitter, maybe they’d also be interested in someone’s personal experience reflecting on the story.
The goal of AnecbotalNYT was to bridge the New York Timescommenting community back into the network of people sharing links to New York Times articles on Twitter. People who might not otherwise pay attention to New York Times comments thus became a new potential audience to engage. And engage it did. One tweet the bot sent received 124 retweets, 291 likes, and 5,374 people clicking on the comment to read it in full. That article was about Cassandra Butts, an Obama-era appointee who died waiting for confirmation from a Republican Senate. AnecbotalNYT’s curated comment for the story struck a chord with liberals, capturing a common sentiment and sharply critical attitude toward a US Senate viewed as playing political games at the expense of individuals like Cassandra. That’s just one example of the kind of engagement the bot can generate. Over the course of April 2017 Twitter users engaged with 57 percent of the 1,191 tweets the bot sent, including some combination of retweets, likes, and replies.
Presenting information via chat interfaces also offers new possibilities for framing that information using the persona of the bot, which can enliven and provide levity to the interaction and make complex material more accessible. It’s here where we truly see the medium start to differentiate itself as something more than a straightforward disseminator of information. One of the more offbeat examples of this approach is a project from the German broadcasting corporation Westdeutscher Rundfunk called “Superkühe” (German for “super cows”). The project followed three cows (Uschi, Emma, and Connie) from three different farms over the course of thirty days in 2017, exposing and contrasting differences in the agricultural production of milk on an organic farm, a family farm, and a factory farm. Daily reports included images, videos, and written content produced by reporters who were following each cow as it gave birth to a new calf and entered into milk production. Sensors placed around (and inside) the cows tracked milk production, health, eating behavior, and activity level.
All of the structured data and content about the cows then fed into a chatbot on Facebook Messenger, which allowed users to interact and chat with a simulation of any of the three cows. By personifying the experiences of each cow and using the chat interface to frame a more intimate encounter, the bot creates an opportunity to empathize with the animal’s experience and learn about animal conditions and treatment relating to different agricultural approaches in a casual and even entertaining format. Instead of reporting about an entity such as a cow, the use of bots creates an opportunity to interact directly with a simulation of that cow, leading to a shift in perspective from third to second person. Consider the possibilities for news storytelling: instead of reading a quote from a source a reporter had interviewed, readers themselves could chat with that source via a bot that simulated responses based on the information the reporter had collected. One advantage might be to draw users in closer to the story and the “characters” of the news.
In some cases bots not only gather information but also process that information to operate as public-facing monitoring and alerting tools. Given the importance of Twitter to the Trump presidency, Twitter bots are routinely oriented toward monitoring Trump-related activity on the platform. For instance, the @TrumpsAlert bot tracks and tweets about the following and unfollowing actions of Trump and his family and inner circle in order to bring additional attention to relationships at the White House. The @BOTUS bot produced by National Public Radio (NPR) had the goal of automatically making stock trades based on monitoring the sentiment of Trump’s tweets when he mentioned publicly traded companies. Another Twitter bot, @big_cases, from USA Todaymonitors major cases in US district courts, including those relating to Trump executive orders. Quartz built a bot called @actual_ransom that monitored the Bitcoin wallets of hackers who had blackmailed people into sending a ransom in order to unlock their computers. The bot, which broke news on Twitter, was the first to report that the hackers had started withdrawing money from the bitcoin wallets. Although none of these monitoring bots is interactive, all do demonstrate the potential of bots to complete the autonomous gathering, analysis, and dissemination circuit in narrowly defined domains.
The ability of bots to monitor aspects of public life and behavior invites examination of how they may contribute to the accountability function of journalism
Bots can also be connected up to streams of data produced by sensors to provide additional monitoring capabilities over time, including of environmental conditions such as air quality. A notable example of a monitoring bot is @GVA_Watcher, which posts to various social media channels when air traffic sensors run by amateur plane-spotters around Geneva’s airport in Switzerland recognize a signal from a plane registered to an authoritarian regime. The bot is intended to draw attention to the travel patterns of authoritarian leaders who may be entering Switzerland for nondiplomatic reasons, such as money laundering.
The ability of bots to monitor aspects of public life and behavior invites examination of how they may contribute to the accountability function of journalism. Can bots help hold public actors accountable for their behavior by drawing more attention to those behaviors on social media platforms?
The attention bots bring to an issue can, at the very least, serve as a constructive starting point for discussion. Take the @NYTAnon bot on Twitter, for example. John Emerson designed the bot for the express purpose of accountability. “It was to kind of put pressure on the Times to be a little stricter about when its sources are or are not anonymous,” he told me. The practice of using anonymous sources by news media is a fraught one, because while it may be justified in some cases in order to protect sources, it also undermines the reader’s ability to evaluate the trustworthiness of the information source on their own. The key is to not overuse anonymous sources or be lax in offering anonymity just because a source is feeling timid. The bot actively monitors all articles published by the New York Times for the use of language relating to the reliance on unnamed or anonymous sources. If an article uses any of 170 different phrases such as “sources say,” “military officials said,” or “requested anonymity,” the bot will excerpt that piece of the article and tweet it out as an image to draw attention to the context in which the New York Timesis using an anonymous source. The initial reaction to the bot included some independent blog posts as well as a post by then-New York Times public editor Margaret Sullivan suggesting that at the very least she and perhaps others in the newsroom were aware the bot was monitoring their use of anonymous sources. Still, despite the NYT’s awareness of the bot’s exposure of its practices, Emerson lamented that he still didn’t know “if it’s changed policy or made reporters think twice about anything.”
To try to answer this question I collected some data on the proportion of New York Timesnews articles that had used any of the 170 terms the bot was tracking over time, both before and after the bot was launched. Did reporters use fewer phrases with respect to anonymous sourcing after the bot started monitoring? The results indicated that there was a slight shift downward in the use of anonymous sources, perhaps as much as 15 percent, in the three months after the bot launched, but that the use of anonymous sources then increased again. There was no clear or definitive signal. I talked to Phil Corbett, the associate managing editor for standards at the New York Timesabout the pattern. According to Corbett they didn’t “detect any major shift” in their use of anonymous sources during that period, but he wasn’t able to firmly refute the possibility of a change either. “I will say that I don’t think much attention was paid to the Anon bot, so that seems to me unlikely to have had much effect. On the other hand, Margaret and some of the other public editors did periodically focus attention on this issue, so that could have had some impact,” Corbett added. The more likely route to accountability here was perhaps not the bot directly, but rather the public editor drawing attention to the issue, which in at least one instance was spurred by the bot when she blogged about it. Bots may not be able to provide enough publicity or public pressure all by themselves. But to be more effective they could be designed to attract attention and cause other media to amplify the issue the bot exposes.
Excerpt adapted from “Automating the News: How Algorithms Are Rewriting the Media,” by Nicholas Diakopoulos (Harvard University Press, 2019). Copyright © 2019 by the President and Fellows of Harvard College. Reprinted with permission from Harvard University Press.